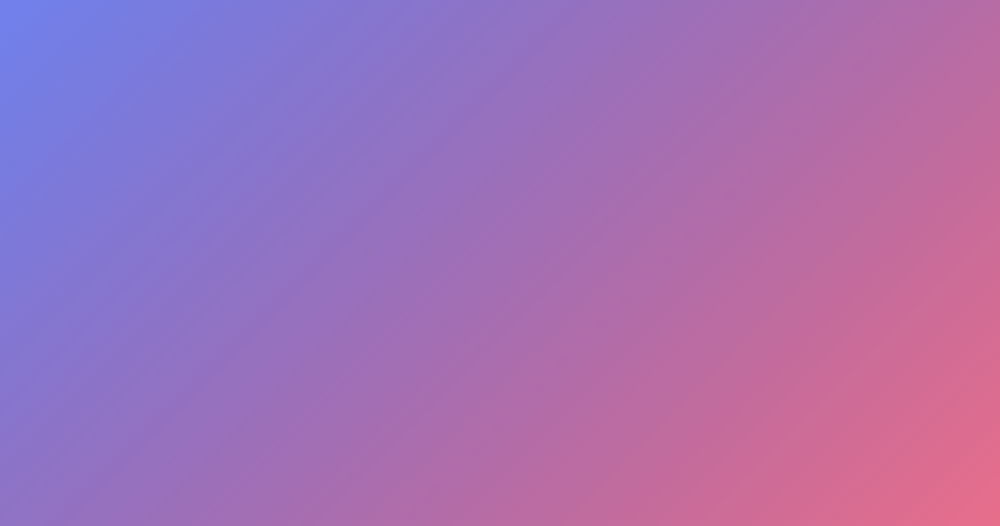
Little Identified Methods to Deepseek
In recent years, it has turn out to be best known as the tech behind chatbots reminiscent of ChatGPT - and DeepSeek - also referred to as generative AI. DeepSeek, doubtless one of the best AI analysis group in China on a per-capita foundation, says the primary factor holding it back is compute. One in all the main features that distinguishes the DeepSeek LLM household from different LLMs is the superior efficiency of the 67B Base model, which outperforms the Llama2 70B Base mannequin in several domains, corresponding to reasoning, coding, arithmetic, and Chinese comprehension. To establish our methodology, we start by developing an expert mannequin tailored to a specific area, equivalent to code, arithmetic, or basic reasoning, using a combined Supervised Fine-Tuning (SFT) and Reinforcement Learning (RL) coaching pipeline. As well as, we carry out language-modeling-based evaluation for Pile-check and use Bits-Per-Byte (BPB) because the metric to guarantee truthful comparison amongst fashions using completely different tokenizers. Note that because of the modifications in our analysis framework over the past months, the performance of DeepSeek-V2-Base exhibits a slight difference from our previously reported outcomes. From the table, we can observe that the MTP strategy constantly enhances the mannequin efficiency on most of the analysis benchmarks. 2) Compared with Qwen2.5 72B Base, the state-of-the-art Chinese open-source model, with only half of the activated parameters, DeepSeek-V3-Base additionally demonstrates remarkable advantages, particularly on English, multilingual, code, and math benchmarks.
As for Chinese benchmarks, apart from CMMLU, a Chinese multi-topic a number of-selection job, DeepSeek-V3-Base also reveals higher efficiency than Qwen2.5 72B. (3) Compared with LLaMA-3.1 405B Base, the largest open-source model with 11 occasions the activated parameters, DeepSeek-V3-Base also exhibits a lot better efficiency on multilingual, code, and math benchmarks. 1) Compared with DeepSeek-V2-Base, because of the enhancements in our mannequin structure, the dimensions-up of the mannequin dimension and coaching tokens, and the enhancement of data quality, DeepSeek-V3-Base achieves considerably higher efficiency as anticipated. Overall, DeepSeek-V3-Base comprehensively outperforms DeepSeek-V2-Base and Qwen2.5 72B Base, and surpasses LLaMA-3.1 405B Base in the majority of benchmarks, essentially becoming the strongest open-source model. As for English and Chinese language benchmarks, deepseek ai-V3-Base reveals competitive or higher efficiency, and is very good on BBH, MMLU-series, DROP, C-Eval, CMMLU, and CCPM. This flexibility allows experts to higher specialize in different domains. To further investigate the correlation between this flexibility and the benefit in model efficiency, we moreover design and validate a batch-clever auxiliary loss that encourages load balance on every coaching batch as an alternative of on every sequence.
In addition, although the batch-sensible load balancing methods present consistent efficiency advantages, in addition they face two potential challenges in effectivity: (1) load imbalance within sure sequences or small batches, and (2) area-shift-induced load imbalance during inference. After a whole lot of RL steps, the intermediate RL model learns to include R1 patterns, thereby enhancing general performance strategically. The experimental results present that, when attaining an identical stage of batch-wise load stability, the batch-clever auxiliary loss may also obtain comparable mannequin efficiency to the auxiliary-loss-free technique. In Table 4, we show the ablation results for the MTP technique. In Table 5, we show the ablation results for the auxiliary-loss-free balancing strategy. In Table 3, we compare the bottom model of DeepSeek-V3 with the state-of-the-artwork open-supply base fashions, including DeepSeek-V2-Base (DeepSeek-AI, 2024c) (our earlier release), Qwen2.5 72B Base (Qwen, 2024b), deepseek and LLaMA-3.1 405B Base (AI@Meta, 2024b). We consider all these fashions with our internal evaluation framework, and be certain that they share the same analysis setting. Under our coaching framework and infrastructures, coaching DeepSeek-V3 on each trillion tokens requires solely 180K H800 GPU hours, ديب سيك which is way cheaper than training 72B or 405B dense models.
The model pre-skilled on 14.Eight trillion "excessive-quality and diverse tokens" (not in any other case documented). The mannequin was pretrained on "a numerous and high-quality corpus comprising 8.1 trillion tokens" (and as is frequent as of late, no different data in regards to the dataset is available.) "We conduct all experiments on a cluster outfitted with NVIDIA H800 GPUs. Upon completing the RL training phase, we implement rejection sampling to curate excessive-quality SFT knowledge for the ultimate model, where the skilled fashions are used as information generation sources. Our last dataset contained 41,160 downside-resolution pairs. DeepSeek has created an algorithm that enables an LLM to bootstrap itself by starting with a small dataset of labeled theorem proofs and create increasingly higher high quality example to fine-tune itself. Model details: The DeepSeek fashions are skilled on a 2 trillion token dataset (break up across largely Chinese and English). Damp %: A GPTQ parameter that impacts how samples are processed for quantisation.
Should you loved this informative article and you want to acquire more details concerning deepseek ai china i implore you to visit our own website.
Reviews