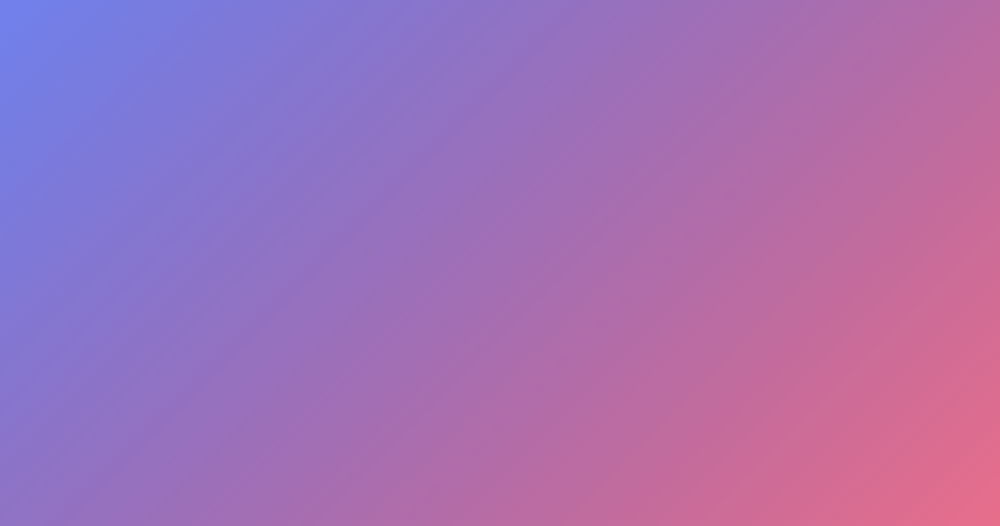
Rules Not to Follow About Deepseek
They are of the identical architecture as deepseek ai LLM detailed beneath. Otherwise you would possibly need a unique product wrapper across the AI model that the larger labs aren't concerned about constructing. The reward mannequin produced reward indicators for each questions with goal but free-kind solutions, and questions without goal solutions (akin to inventive writing). A number of questions comply with from that. One in all the key questions is to what extent that data will end up staying secret, each at a Western firm competition level, in addition to a China versus the remainder of the world’s labs stage. But they find yourself persevering with to only lag just a few months or years behind what’s happening within the main Western labs. Ok so I've really discovered a few issues concerning the above conspiracy which does go against it, considerably. There’s a very prominent instance with Upstage AI final December, where they took an concept that had been in the air, applied their own name on it, deepseek and then revealed it on paper, claiming that idea as their very own. Therefore, it’s going to be exhausting to get open source to build a better mannequin than GPT-4, just because there’s so many issues that go into it.
That was shocking because they’re not as open on the language mannequin stuff. You can see these concepts pop up in open supply where they attempt to - if people hear about a good idea, they try to whitewash it after which brand it as their own. Why this matters - lots of notions of control in AI coverage get harder in the event you need fewer than 1,000,000 samples to transform any mannequin into a ‘thinker’: Probably the most underhyped part of this release is the demonstration which you can take models not trained in any sort of main RL paradigm (e.g, ديب سيك Llama-70b) and convert them into powerful reasoning models using simply 800k samples from a strong reasoner. Shawn Wang: I would say the main open-source fashions are LLaMA and Mistral, and each of them are highly regarded bases for creating a number one open-supply model. OpenAI, DeepMind, these are all labs which are working towards AGI, I'd say.
You can’t violate IP, but you may take with you the data that you just gained working at a company. Large language fashions (LLMs) are highly effective instruments that can be used to generate and perceive code. We also can speak about what among the Chinese firms are doing as properly, which are fairly attention-grabbing from my point of view. Why this issues: First, it’s good to remind ourselves that you can do an enormous quantity of useful stuff with out slicing-edge AI. Whereas, the GPU poors are usually pursuing extra incremental adjustments based mostly on techniques which are recognized to work, that may improve the state-of-the-artwork open-supply models a reasonable quantity. The closed fashions are nicely ahead of the open-supply models and the gap is widening. It’s one model that does every thing really well and it’s wonderful and all these different things, and will get closer and nearer to human intelligence. Thus far, despite the fact that GPT-4 completed coaching in August 2022, there is still no open-supply mannequin that even comes near the unique GPT-4, a lot less the November sixth GPT-four Turbo that was launched.
That's even better than GPT-4. The open-supply world has been really nice at helping companies taking some of these models that are not as capable as GPT-4, but in a really slim area with very particular and unique data to your self, you can also make them better. You can go down the checklist and wager on the diffusion of data by humans - pure attrition. They do take information with them and, California is a non-compete state. That does diffuse data quite a bit between all the large labs - between Google, OpenAI, Anthropic, no matter. But these appear extra incremental versus what the big labs are prone to do when it comes to the massive leaps in AI progress that we’re going to likely see this yr. While the 2 firms are each developing generative AI LLMs, they've different approaches. While the MBPP benchmark consists of 500 problems in just a few-shot setting.
If you have any thoughts pertaining to exactly where and how to use deep seek, you can contact us at the web site.
Reviews