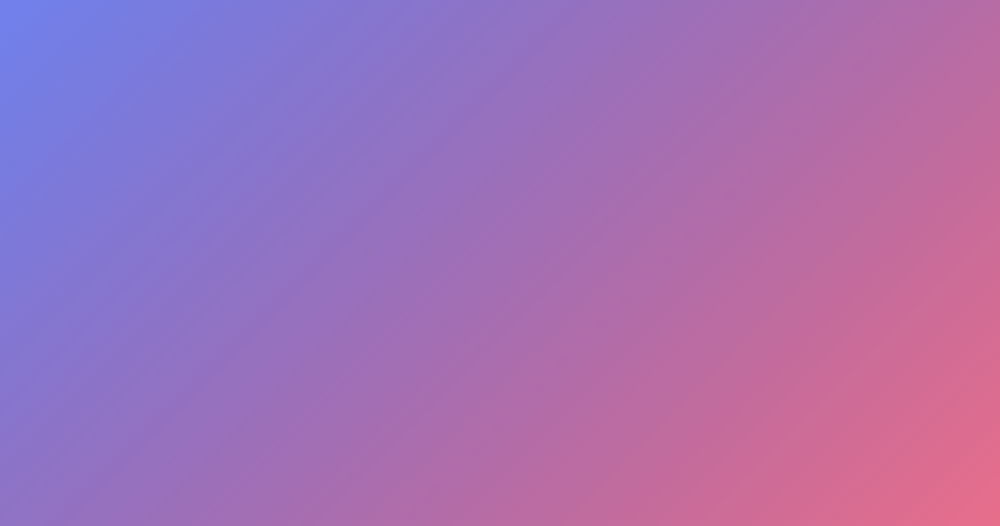
Six Mistakes In Deepseek That Make You Look Dumb
DeepSeek is revolutionizing healthcare by enabling predictive diagnostics, personalized medicine, and drug discovery. We examined 4 of the top Chinese LLMs - Tongyi Qianwen 通义千问, Baichuan 百川大模型, deepseek ai 深度求索, and Yi 零一万物 - to evaluate their means to answer open-ended questions about politics, legislation, and history. While it’s not essentially the most practical model, DeepSeek V3 is an achievement in some respects. Compared with free deepseek-V2, we optimize the pre-coaching corpus by enhancing the ratio of mathematical and programming samples, whereas expanding multilingual protection beyond English and Chinese. Throughout the pre-training state, training DeepSeek-V3 on every trillion tokens requires only 180K H800 GPU hours, i.e., 3.7 days on our own cluster with 2048 H800 GPUs. For the MoE part, every GPU hosts just one knowledgeable, and 64 GPUs are answerable for internet hosting redundant experts and shared specialists.
Evaluation particulars are here. However, this trick could introduce the token boundary bias (Lundberg, 2023) when the model processes multi-line prompts without terminal line breaks, significantly for few-shot evaluation prompts. D is ready to 1, i.e., in addition to the precise subsequent token, every token will predict one extra token. Under this configuration, DeepSeek-V3 contains 671B complete parameters, of which 37B are activated for each token. In the present course of, we have to read 128 BF16 activation values (the output of the previous computation) from HBM (High Bandwidth Memory) for quantization, and the quantized FP8 values are then written again to HBM, solely to be read once more for MMA. This significantly reduces the dependency on communication bandwidth compared to serial computation and communication. We aspire to see future distributors developing hardware that offloads these communication tasks from the valuable computation unit SM, serving as a GPU co-processor or a network co-processor like NVIDIA SHARP Graham et al.
Given the substantial computation concerned in the prefilling stage, the overhead of computing this routing scheme is nearly negligible. Furthermore, in the prefilling stage, to improve the throughput and hide the overhead of all-to-all and TP communication, we simultaneously course of two micro-batches with similar computational workloads, overlapping the attention and MoE of one micro-batch with the dispatch and mix of one other. However, the current communication implementation depends on expensive SMs (e.g., we allocate 20 out of the 132 SMs obtainable in the H800 GPU for this function), which can limit the computational throughput. The high-load specialists are detected based on statistics collected during the online deployment and are adjusted periodically (e.g., each 10 minutes). We achieve these three targets with out compromise and are committed to a focused mission: bringing flexible, zero-overhead structured generation all over the place. Within the training strategy of DeepSeekCoder-V2 (DeepSeek-AI, 2024a), we observe that the Fill-in-Middle (FIM) strategy does not compromise the subsequent-token prediction capability while enabling the mannequin to precisely predict center text primarily based on contextual cues. The pretokenizer and training data for our tokenizer are modified to optimize multilingual compression efficiency. However, critics are involved that such a distant-future focus will sideline efforts to tackle the various pressing moral points going through humanity now.
The information that TSMC was mass-producing AI chips on behalf of Huawei reveals that Nvidia was not fighting in opposition to China’s chip industry however somewhat the mixed efforts of China (Huawei’s Ascend 910B and 910C chip designs), Taiwan (Ascend chip manufacturing and CoWoS advanced packaging), and South Korea (HBM chip manufacturing). Alternatively, a near-reminiscence computing approach could be adopted, where compute logic is placed close to the HBM. It will possibly have important implications for functions that require looking out over a vast space of attainable solutions and have tools to confirm the validity of mannequin responses. Addressing the mannequin's efficiency and scalability would be vital for wider adoption and real-world functions. For the MoE part, we use 32-method Expert Parallelism (EP32), which ensures that each knowledgeable processes a sufficiently large batch dimension, thereby enhancing computational effectivity. This approach ensures that errors remain inside acceptable bounds while maintaining computational effectivity. Also, our information processing pipeline is refined to minimize redundancy whereas sustaining corpus variety. This view is supported by macro-level data from studies throughout national borders. Large language models (LLM) have shown spectacular capabilities in mathematical reasoning, however their application in formal theorem proving has been restricted by the lack of coaching data.
If you have any queries about where by and how to use ديب سيك مجانا, you can get in touch with us at the web-site.
Reviews